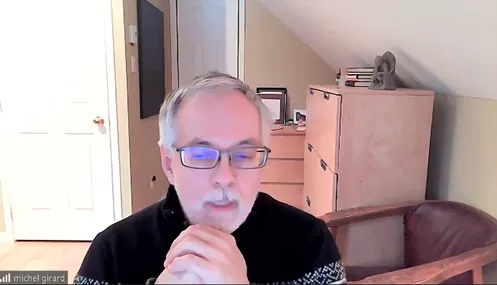
Mastering Digital Technologies, Governance, and Value Chains Hosted by Michel Girard – IMI BIGDataAIHUB Seminar Series
Companies are rapidly moving from analog to digital operations through tools such as artificial intelligence (AI), robotics process automation (RPA), bots, E-commerce, cloud platforms, and Industry 4.0. The drive to digital can be attributed to various causes, such as the COVID-19 Pandemic, an aging workforce, supply-chain constraints, competition and cost-reduction strategies, and the Great Resignation. However, as organizations adopt digital technologies, they find data quality, access, and analytics issues. At present, there is a lack of adequate systems of control compounded with limited strategy and governance. These limitations are creating mistrust amongst developers, senior management teams and boards, and the general public. This decline in trust in digital tech, particularly AI, is found globally (See the 2021 Edelman Trust Barometer found here).
Governance for AI in Products versus Services
This leaves us with the obvious question: how do we inject trust into the data and its outcomes? The answer to this question lies between a tricky balance of advancing innovation and upholding safety. One option is to have third-party verifiers vouch for the design, production, and the use of AI in tangible products. For AI in services, however, a different approach is needed, namely assurance engagements. Assurance engagements will help establish trust, but they will never be able to say with 100% accuracy if something is safe within a service offering; there will always be risks.
The Centre for International Governance Innovation has developed five principles for standardizing products in AI. These principles include accuracy, explainability, resiliency, safety, and reliability. Michel Girard argues this is a good path forward, but it is more tricky to establish principles surrounding the use of AI in services. In the case of services, value-laden criteria are also necessary. For instance, objectivity —where AI systems are devoid of prejudice or bias against individuals or groups—is a must. Furthermore, inclusive growth, sustainable development, and well-being are also requisites. Here, AI systems should be beneficial for people and the planet, reflecting fundamental values of fairness and privacy.
Resources for Data Ethics and Governance
Many organizations are starting to think about data governance. Michel provided the example of IEEE Ethically Aligned Design Initiative, where over 35 standards have been created to help data scientists think through ethical issues associated with their work, see more here. Another resource is the Data Ethics Canvas, where implications can be considered from data sources down to individual actions, find this resource here. Digital governance standards are also being developed in Canada by the CIO Strategy Council, find more information here.
Moving Forward in a Digital World
The path toward digitization has five inevitable steps:
1. Understand Data Value Chains
A) Data Collection / Gathering
The emphasis must be on gathering high-quality data, where data labelling and data attributes are understood. Currently, there are no standards in place to assess and report on the quality of data. This process is likely best managed by a data engineer who owns the cleaning, collection, and grading process. Where cleaning is the process of using algorithms to ensure the data is usable, collection involves processes to gather the data, and grading is a system to assess the quality of the data collected.
B) Data Access / Sharing
Data sharing should be managed by a data controller who will evaluate the internal use, external use, and potential jurisdictional issues. A data controller is someone who will be accountable to find the data sources and apply filters so those requesting data have access to the right data, at the right place, at the right time. The data controller should ask who should have access to the data? Can the origins and quality of the data be validated? What changes are affecting the use of data/insights? Rules for data access are very important to increase trust and reduce risk.
C) Data Analytics / Insights
A data scientist can help provide data insights through measurement, modelling, and analytics.
2. Set Priorities Through a Digitization Strategy
Once the data value chain is understood, the organization is ready to create a digitization strategy. A digitization strategy is critical to success. Start small at first to ensure your digitization strategy is aligned with the overall strategic direction of the organization. Set priorities because resources are scarce, have a budget, and determine how results will be gained.
3. Establish Clear Rules for Data Sharing / Reuse
Formulate a data policy to outline how the organization’s data will be managed. Ensure to provide clarity surrounding: common definitions, data ownership/ IP/ copyright, data tagging and tracking, compliance with relevant privacy/ human rights/ digital identity regulations, safe use/ ethics/ trust/ biases /explainability, data residency, accountability for data collection/ access/ analytics/ assurance/ risk management. See this article on elements of a corporate data policy here.
4. Communicate Results and Foster Trust
The risk of data misuse is high! Data must be managed properly.
5. See Data as an Asset
Currently, there is no methodology to determine the value of our data sets. With increasing regulations, it is possible that data has a negative value given the risks of misuse and leakage. Additional insights on the worth of data can be found in this article.
This is only a high-level summary of the incredibly rich insights shared by Michel Girard during the third seminar in the 2022-2023 IMI BIGDataAIHUB’s Seminar Series. Michel emphasized the immense opportunities available in the space. A team of experts is needed to ensure the integrity of data and its use within organizations.